

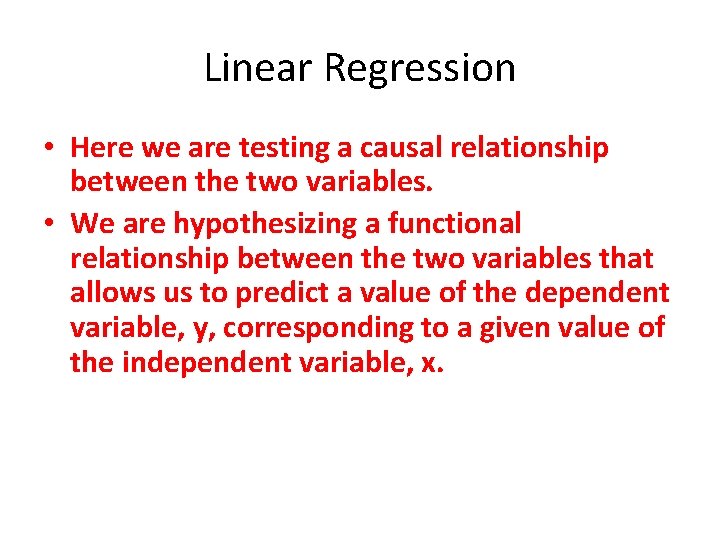
However, this is only one of a number of causality criteria at the population level for determining whether a vaccine may have caused a particular AE (others include temporal relationship, dose-response relationship, consistency of evidence, specificity, biological plausibility, and coherence). These DMAs all share the same objective: to estimate the strength of association. In this study, we focus on the GlaxoSmithKline (GSK) vaccines SRD containing spontaneously reported adverse events (AEs) following immunization by a GSK vaccine. Over time, the use of these DMAs extended to SRDs held by drug and vaccine manufacturers. These DMAs were first developed for screening the SRDs held by regulatory authorities: the Empirical Bayes Geometric Mean (EBGM) for the Food and Drug Administration SRD, the information component (IC) for the World Health Organization (WHO) SRD, the proportional reporting ratio (PRR) for the UK SRD, and the reporting odds ratio for the Netherlands Pharmacovigilance Foundation Lareb SRD. This measure of disproportionality offers a proxy of the strength of association between a product and an event while accounting for the absence of exposure data characteristics of spontaneous data. The majority of these algorithms detect product–event pairs (P–Es) presenting a disproportionate number of reports compared with the expected number from other/all products and other/all events within the same SRD. FormalParaĭata mining algorithms (DMAs) have been developed for screening spontaneous report databases (SRDs). Logistic regression combines and weights these causality criteria based on their respective ability to predict known safety issues. Logistic regression allows estimation of the probability of a vaccine–event pair being an ARFI using two causality criteria at the population level assessed in spontaneous report data: the strength of association (disproportionality measure) and temporality (TTO distribution tests).
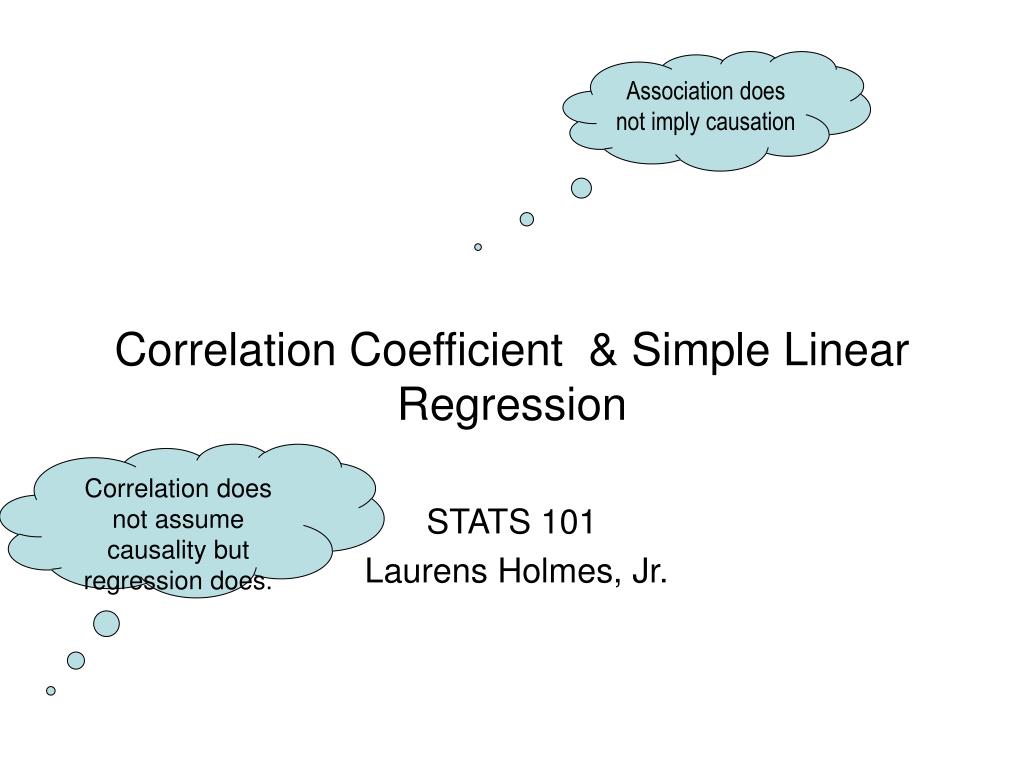
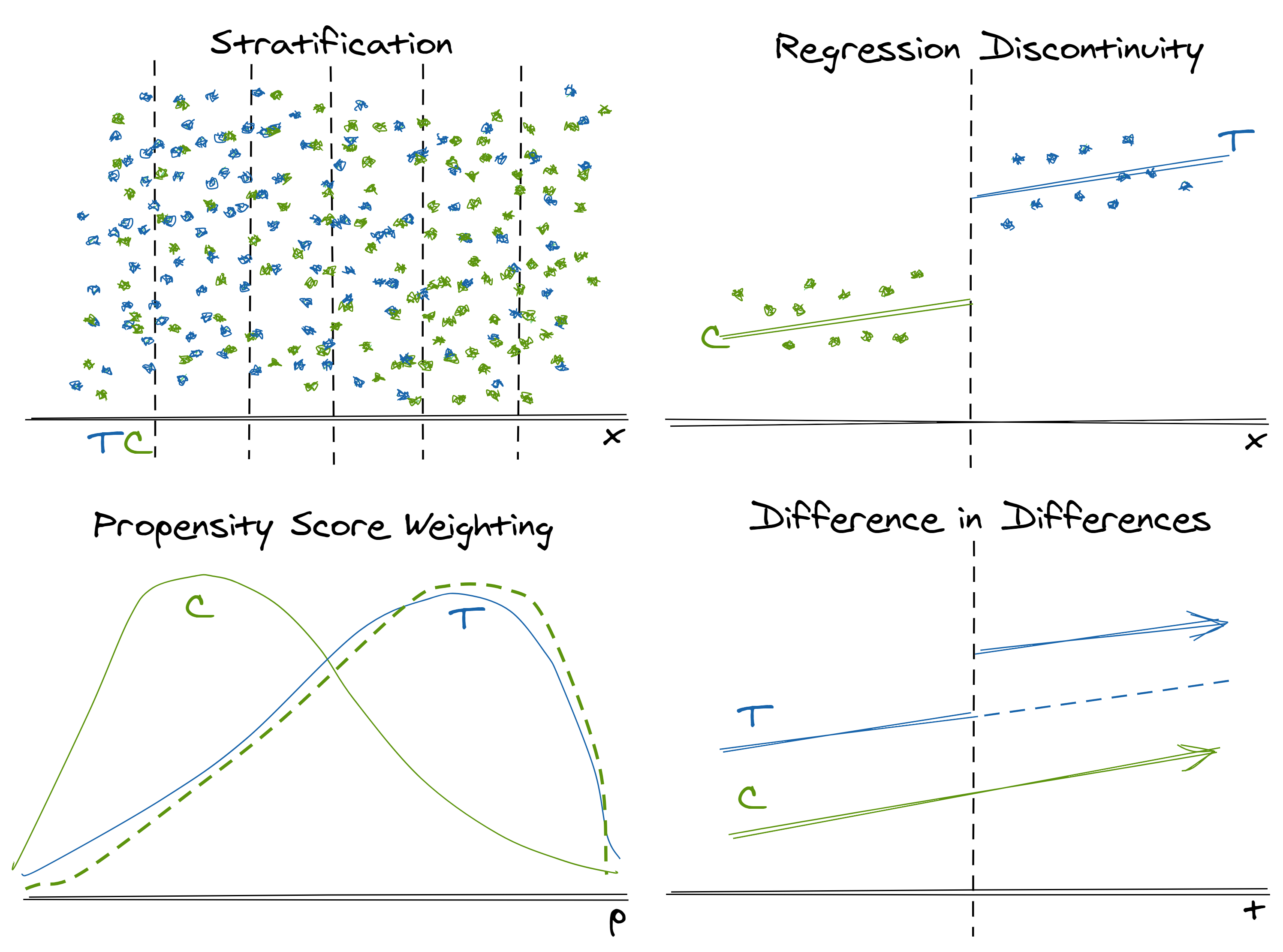
The p value of the ‘between vaccines’ KS test was the most significant predictive factor. Model 3, using two quantified causality criteria, provided the best performance for all measures. The performance of the regressions (model fit statistics, calibration, and discrimination) was measured on 100 bootstrap samples. Three logistic regressions were built to predict ARFIs based on different combinations of the proportional reporting ratio (PRR disproportionality measure) and two Kolmogorov–Smirnov (KS) tests (‘between vaccines’ and the ‘between events’) of TTO distribution: model 1, using the PRR estimate and its 95 % lower confidence interval (CI) limit model 2, using the p values of the two KS tests and model 3, using the PRR (point estimate and lower CI limit) and both KS tests. We used spontaneous reports of adverse events from eight vaccines and their labels as proxies for ARFIs. We evaluated the use of logistic regression to model the probabilities of spontaneously reported vaccine–event pairs being adverse reactions following immunization (ARFI), using disproportionality and unexpectedness of time-to-onset (TTO) distributions as predictive variables and the presence of events in the global product information as a dependent variable.
